We rely on the search engine to find information every day, but what if there was a better way?
Instead of manually collecting details from many sources, AI agents can work for you heavy lifting.
They do not just get information. They analyze, systematic and privatize it in real time.
This article shows:
- How AI agents help businesses to create more individual customer experience.
- Major components and outlines behind AI-operated agents.
- How multi-agent systems can cooperate to solve complex tasks.
From information recovery to intelligent problem
AI agents represent a fundamental change of how we interact with AI.
As brands, we are moving beyond passive information recovery-to make a slow procedure-conversion problem-solution of manually collecting data from different websites, where multimodal data is original for a favorite interface in real time Customs from
Imagine a world where many independent AI agents collaborate to complete complex workflows.
Industry experts estimate significant changes due to AI agents. What they have to say here:
- Satya Nadella: AI agents will estimate the needs of the user and basically help.
- Bill Gates: The most important software transformations have been running since AI agent graphical user interface.
- Jensen Huang: IT departments are managing AI agents in the way human resources manage employees.
- Jeff Bezos: AI agents act as a digital copillot, increasing daily interactions.
- Gartner: The quantity of the search engine will decrease by 25% by 2026 as AI chatbots and virtual agents will revolutionize customer interactions.
Today, brands have an important opportunity to benefit AI agents as comrades from the intelligent virtual team, making businesses capable of providing hyper-parsonal experiences.
As the AI agent and technology develops, we are moving away from the efforts taking at the time of collecting information manually.
In the future, the AI agents will interact with each other, collect relevant data, arrange it to match it with users preferences, and distribute it originally – to create a sharp and more efficient experience.
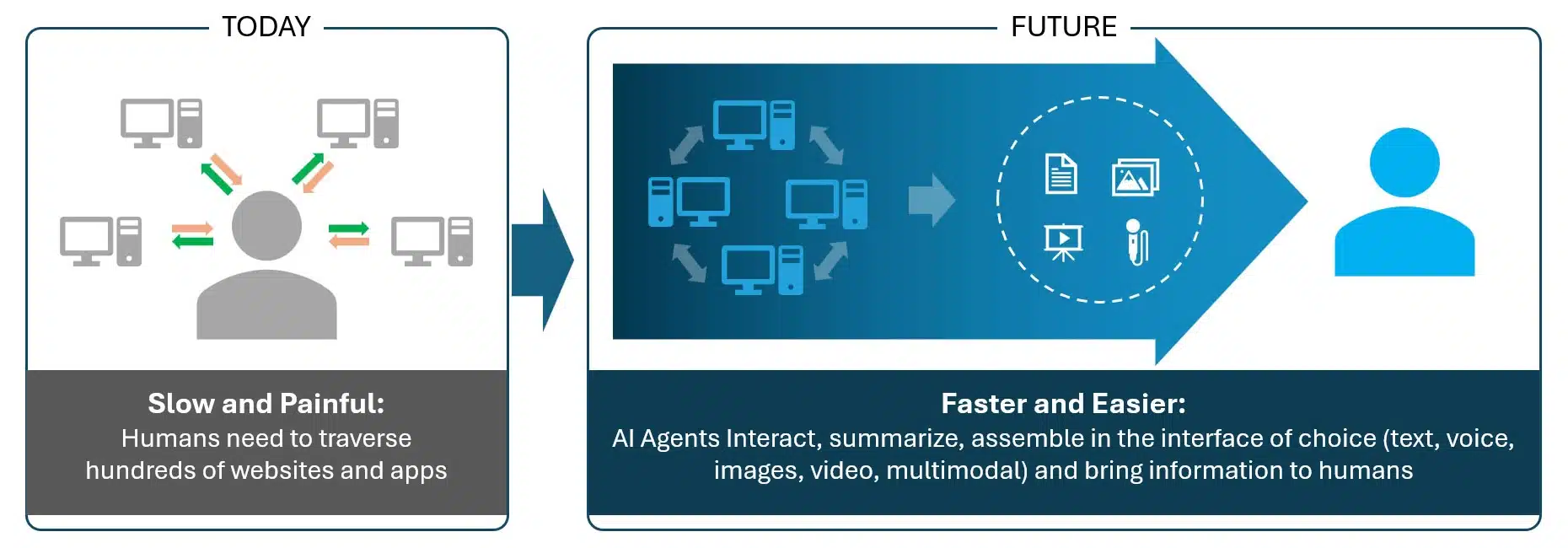
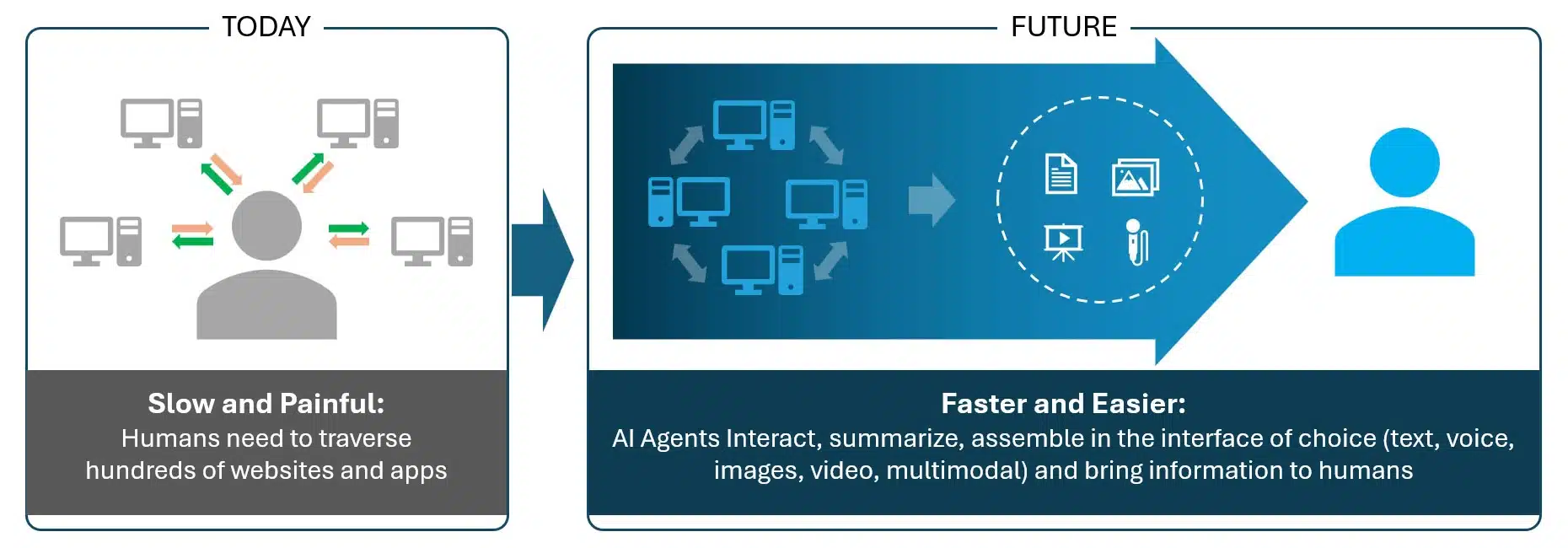
Deep excavation: AI and marketing mastered: a starting guide
To understand how AI agents provide these intelligent, real -time experiences, we need to break their main components.
Let's find out the anatomy of AI agents and how each layer contributes to their functionality.
Anatomy
AI agents are designed to increase the capabilities of LLM by incorporating additional functionality.
There are four layers of agents:
- Foundation layer.
- application layer.
- Management layer.
- Data layer.
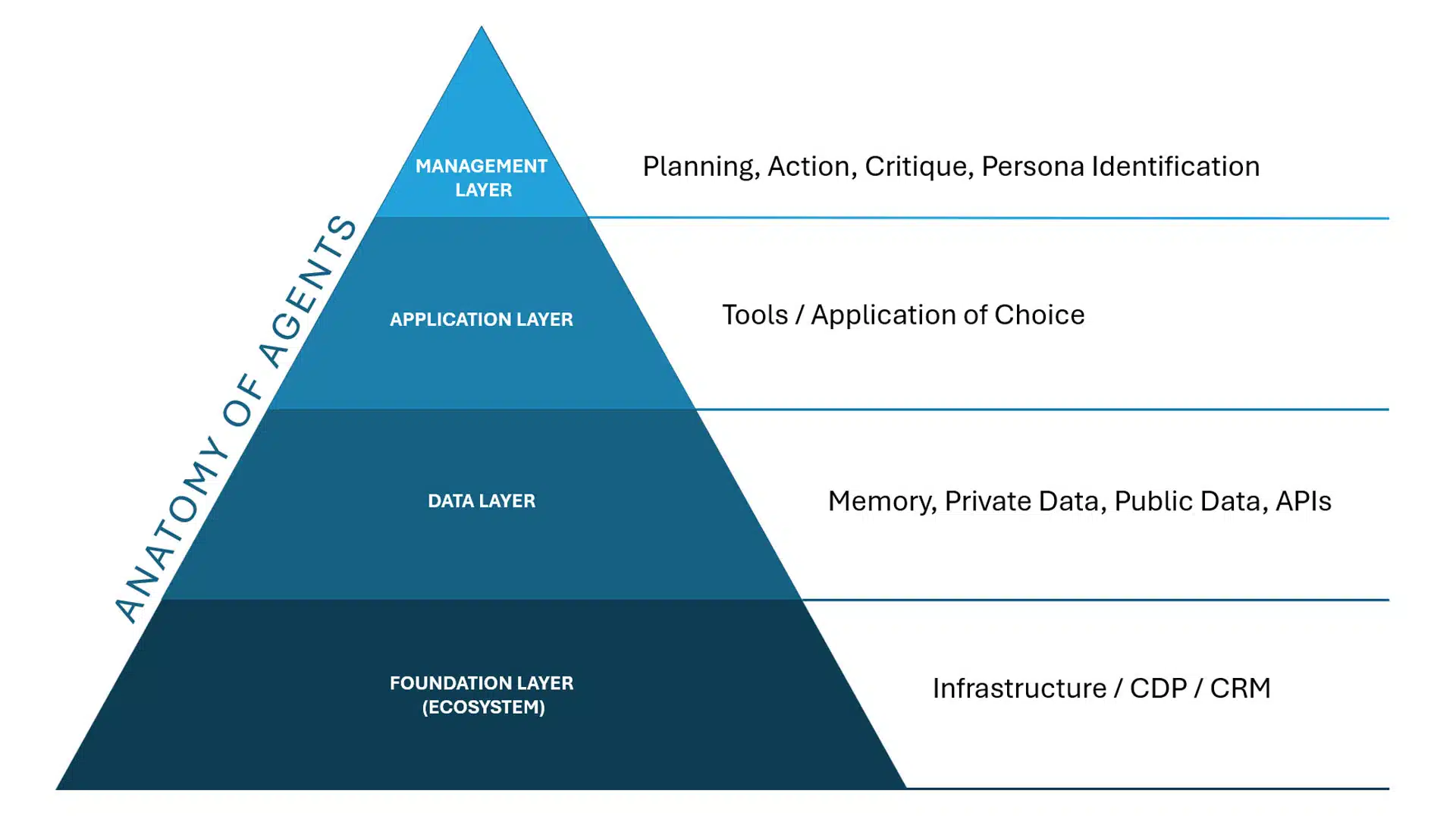
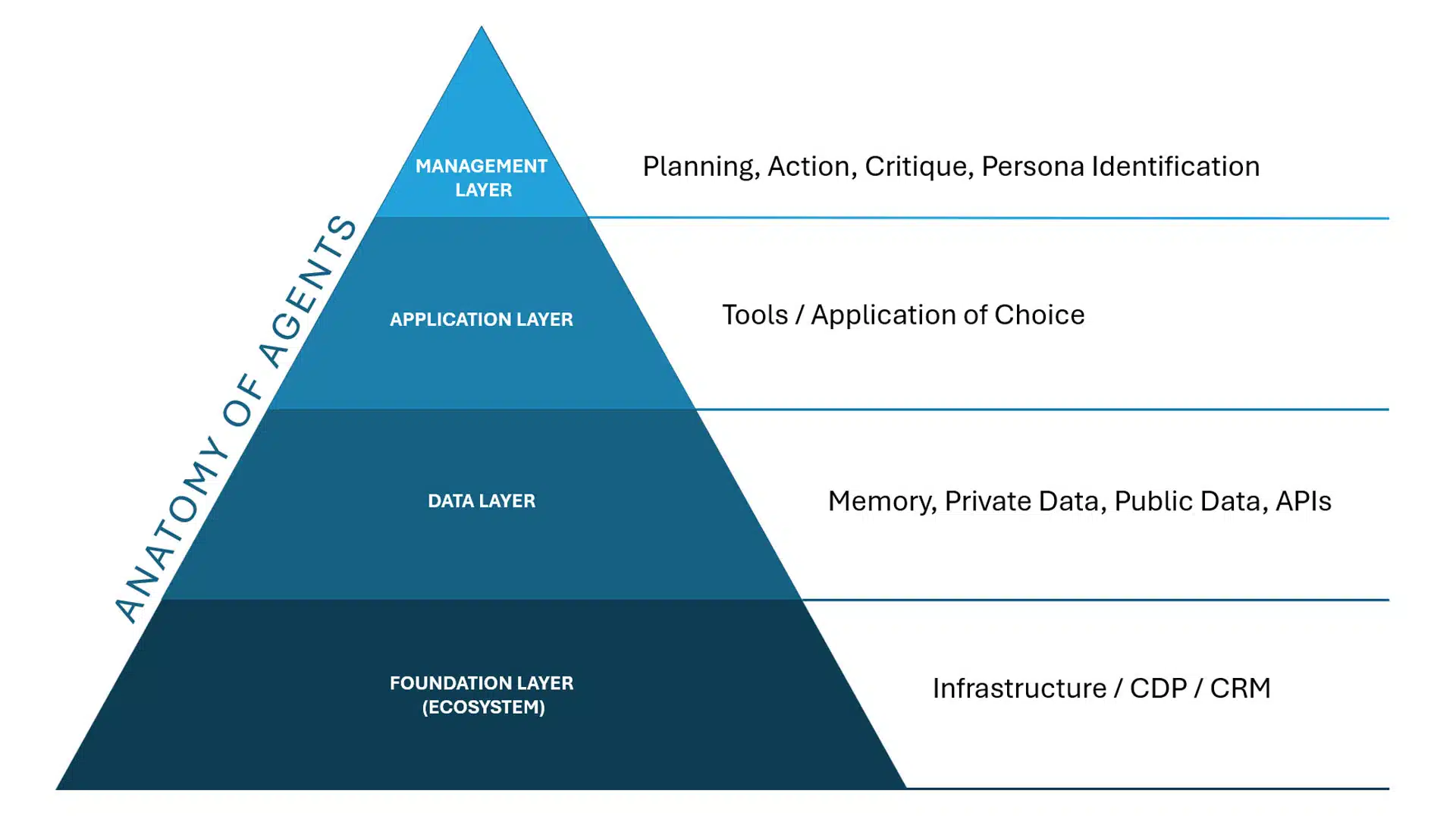
An AI agent usually consists of the following components:
- Memory: Store the previous interaction and response to provide relevant relevant reactions. The memory data lives in the layer.
- Equipment/Forum: Removes real -time data and interacts with the internal database. Selected equipment and platform are part of the application layer.
- Plan: Uses logic techniques to break complex functions into simple stages.
- Work: Execute tasks based on insight from LLM and other sources.
- Criticism: The accuracy provides a response loop for actions based on cases of various use to ensure.
- Persona: Adapt to various roles such as research assistants, content writers or customer aid agents.
The management layer has planning, function, criticism and personality identity.
Fraudline for the manufacture of AI agents
There are many framework available for the construction of AI agents and multi-agent systems, each catering for a different requirement:
- Autogen (Microsoft): The conversion focuses on AI and automation.
- Krevai: Roll-playing agents were designed for effectively.
- Langgraph: Structures agent interaction in a graph-based model.
- Herd (openi): Mainly for educational purposes.
- Langchen: A popular structure enables AI agents to work with LLM and other devices.
Each platform provides unique benefits based on the case, scalability and complexity.
Multi-agent AI system and their importance
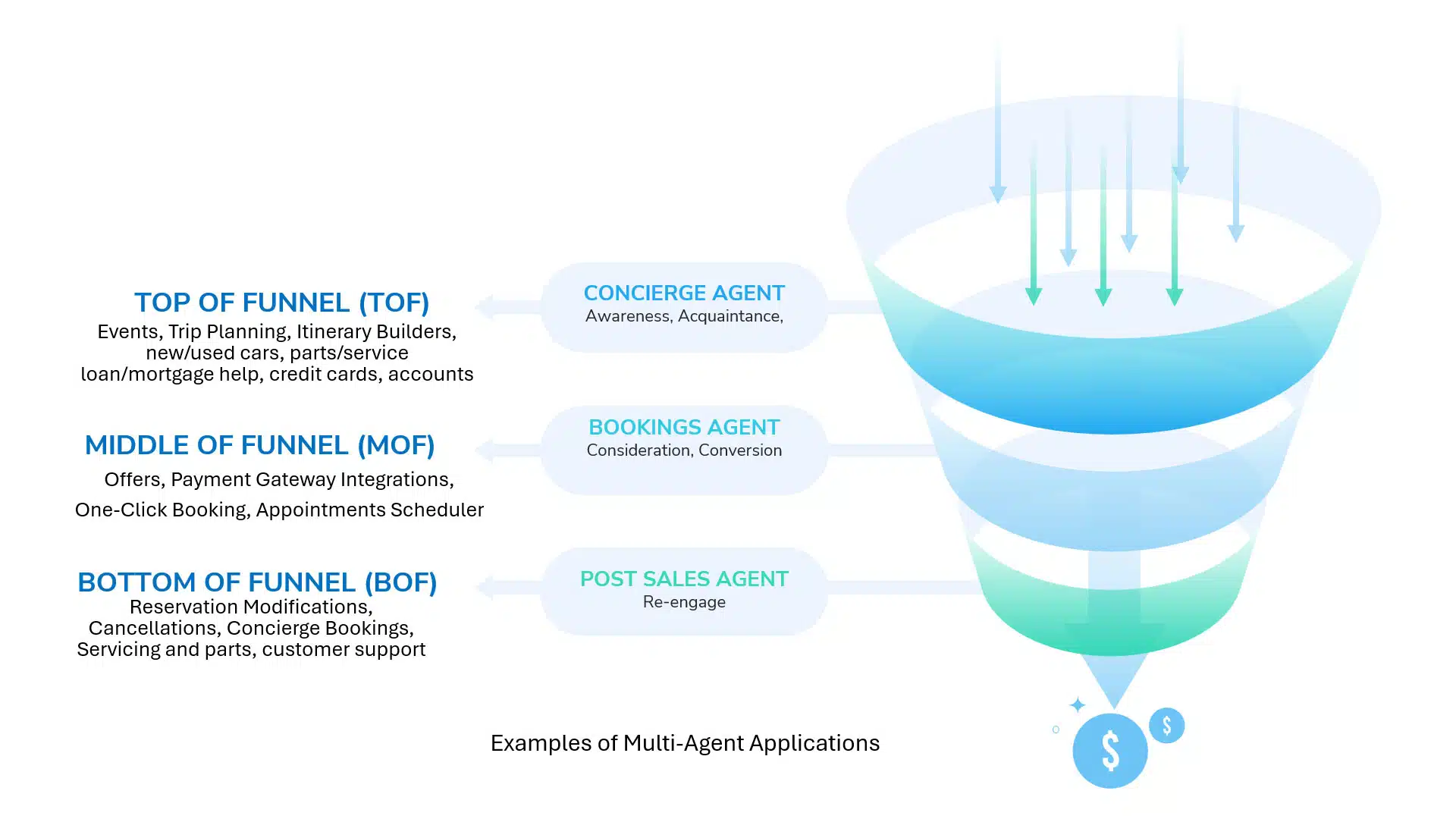
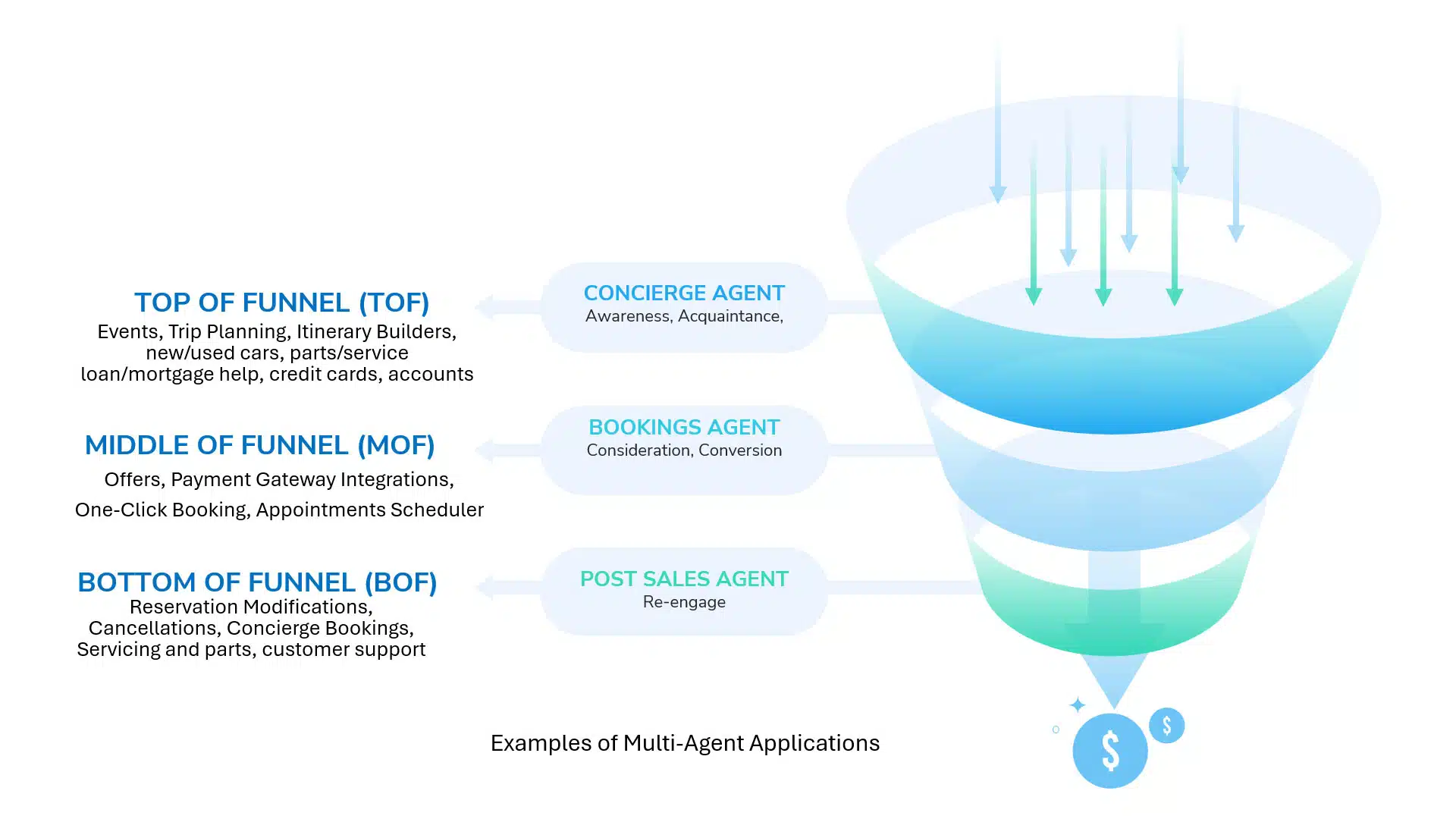
A multi-agent system consists of several AI agents that are originally working, each associately performs a different task to solve problems.
These systems are particularly useful for handling complex scenarios where a single AI agent can struggle.
Below is a simple example of a multi-agent system:
- Querry Processing Agent: Breakes the question into many parts.
- Recovery Agent: Receives relevant data from internal sources.
- Verification Agent: The brand verification the response against various parameters such as voice voice and query intentions.
- Formating Agent: Response properly structures.
This structured approach to distribute responsibilities among agents ensures more accurate and intelligent reactions by reducing errors.
Before finding out how AI agents provide real -time privatization, let's see why traditional methods are not enough now.
Deep excavation: AI adaptation: how to adapt your content for AI search and agents
Why AI-Training Privatization is necessary
As data availability declines and user's expectations increase, businesses can no longer rely on traditional methods to understand the intentions of the customer.
Away from the third-party cookies, the rise of zero-click materials, and real-time demand, analogy experiences have made AI-driven personalization a requirement.
AI enables businesses to analyze behavior, predict intentions and provide dynamic, personal experience-from poz and social to email and on-site interactions.
Unlike static privatization, AI, in real time, ensures relevance at each customer touchpoint.
With traditional strategies lose effectiveness, the AI agents provide a more clever, more scalable way to attach and convert the audience.
Deep digging: How to promote your marketing revenue with privatization, connectivity and data
Finding personal experience with search and chat agents
Modern websites are no longer a size-fit-all. They provide immersive experience in line with each visitor's intention.
AI agents enable it through two major approaches:
Search agent
Traditional site discoveries rely on keywords and filters, with limitations with multimodal discoveries (such as voice or scene) and long-tail queries.
They also require more user clicks, increasing the possibility of discovery abandonment.
The AI-managed search agents exceeded these challenges by providing more comfortable and skilled on-site search experiences.
Chat agent
The initial AI chatbots responded using pre-demogogoged scripts or existing website content.
Today, advanced chat agents provide personal experience using audience data. They can:
- Create a detailed user profile.
- Understand the user's intentions by analyzing historical interaction and purchase data.
- Learn from equal conversation to ask relevant follow -up questions.
- Customize experiences at the site in real time depending on the user behavior.
- Inform the cross-channel marketing strategies-such as using the insight collected from email, social, payment and retiring-user interactions.
AI agents also provide industry-specific privatization. Can apply brands:
- Digital Marketing Automation Agent.
- Customer aid chat agent.
- Special solutions, such as:
- Financial risk evaluation agent.
- Automotive inventory management agent.
Privatization
Many businesses still consider privatization to be alternative.
In fact, without individual experiences, traffic and conversion will decline, which requires more expenses to attract, attach and convert visitors such as high marketing costs and low ROIs.
To improve efficiency, AI-manual privatization provides a scalable, intelligent and adaptive solution.
Deep excavation: Hyper-Personalization in PPC: Using data to give a series of advertising experiences
Authors contributing are invited to create materials for the search engine land and are chosen for their expertise and contribution to the search community. Our contributors work under the supervision of editorial staff and contribute to quality and relevance for our readers. The opinions they express are their own.